Difference between revisions of "Transformer"
m (→Transformers Course | HuggingFace) |
m |
||
(14 intermediate revisions by the same user not shown) | |||
Line 21: | Line 21: | ||
* [[Large Language Model (LLM)]] ... [[Large Language Model (LLM)#Multimodal|Multimodal]] ... [[Foundation Models (FM)]] ... [[Generative Pre-trained Transformer (GPT)|Generative Pre-trained]] ... [[Transformer]] ... [[GPT-4]] ... [[GPT-5]] ... [[Attention]] ... [[Generative Adversarial Network (GAN)|GAN]] ... [[Bidirectional Encoder Representations from Transformers (BERT)|BERT]] | * [[Large Language Model (LLM)]] ... [[Large Language Model (LLM)#Multimodal|Multimodal]] ... [[Foundation Models (FM)]] ... [[Generative Pre-trained Transformer (GPT)|Generative Pre-trained]] ... [[Transformer]] ... [[GPT-4]] ... [[GPT-5]] ... [[Attention]] ... [[Generative Adversarial Network (GAN)|GAN]] ... [[Bidirectional Encoder Representations from Transformers (BERT)|BERT]] | ||
+ | * [[State Space Model (SSM)]] ... [[Mamba]] ... [[Sequence to Sequence (Seq2Seq)]] ... [[Recurrent Neural Network (RNN)]] ... [[(Deep) Convolutional Neural Network (DCNN/CNN)|Convolutional Neural Network (CNN)]] | ||
* [[Natural Language Processing (NLP)]] ... [[Natural Language Generation (NLG)|Generation (NLG)]] ... [[Natural Language Classification (NLC)|Classification (NLC)]] ... [[Natural Language Processing (NLP)#Natural Language Understanding (NLU)|Understanding (NLU)]] ... [[Language Translation|Translation]] ... [[Summarization]] ... [[Sentiment Analysis|Sentiment]] ... [[Natural Language Tools & Services|Tools]] | * [[Natural Language Processing (NLP)]] ... [[Natural Language Generation (NLG)|Generation (NLG)]] ... [[Natural Language Classification (NLC)|Classification (NLC)]] ... [[Natural Language Processing (NLP)#Natural Language Understanding (NLU)|Understanding (NLU)]] ... [[Language Translation|Translation]] ... [[Summarization]] ... [[Sentiment Analysis|Sentiment]] ... [[Natural Language Tools & Services|Tools]] | ||
− | * [[Assistants]] ... [[Personal Companions]] ... [[ | + | * [[Agents]] ... [[Robotic Process Automation (RPA)|Robotic Process Automation]] ... [[Assistants]] ... [[Personal Companions]] ... [[Personal Productivity|Productivity]] ... [[Email]] ... [[Negotiation]] ... [[LangChain]] |
* [[Excel]] ... [[LangChain#Documents|Documents]] ... [[Database|Database; Vector & Relational]] ... [[Graph]] ... [[LlamaIndex]] | * [[Excel]] ... [[LangChain#Documents|Documents]] ... [[Database|Database; Vector & Relational]] ... [[Graph]] ... [[LlamaIndex]] | ||
+ | * [[What is Artificial Intelligence (AI)? | Artificial Intelligence (AI)]] ... [[Generative AI]] ... [[Machine Learning (ML)]] ... [[Deep Learning]] ... [[Neural Network]] ... [[Reinforcement Learning (RL)|Reinforcement]] ... [[Learning Techniques]] | ||
+ | * [[Conversational AI]] ... [[ChatGPT]] | [[OpenAI]] ... [[Bing/Copilot]] | [[Microsoft]] ... [[Gemini]] | [[Google]] ... [[Claude]] | [[Anthropic]] ... [[Perplexity]] ... [[You]] ... [[phind]] ... [[Ernie]] | [[Baidu]] | ||
* [[Sequence to Sequence (Seq2Seq)]] | * [[Sequence to Sequence (Seq2Seq)]] | ||
* [[Recurrent Neural Network (RNN)]] | * [[Recurrent Neural Network (RNN)]] | ||
* [[Long Short-Term Memory (LSTM)]] | * [[Long Short-Term Memory (LSTM)]] | ||
+ | * [[Autoencoder (AE) / Encoder-Decoder]] | ||
+ | * [[T5|T5 (Text-To-Text Transfer Transformer) models]] | ||
* [[Memory Networks]] | * [[Memory Networks]] | ||
− | |||
− | |||
* [[Google]] [[Transformer-XL]] ...T5-XXL ...[http://venturebeat.com/2021/01/12/google-trained-a-trillion-parameter-ai-language-model/ Google trained a trillion-parameter AI language model | Kyle Wiggers - VB] | * [[Google]] [[Transformer-XL]] ...T5-XXL ...[http://venturebeat.com/2021/01/12/google-trained-a-trillion-parameter-ai-language-model/ Google trained a trillion-parameter AI language model | Kyle Wiggers - VB] | ||
* [http://jalammar.github.io/illustrated-transformer/ The Illustrated Transformer | Jay Alammar] | * [http://jalammar.github.io/illustrated-transformer/ The Illustrated Transformer | Jay Alammar] | ||
Line 37: | Line 40: | ||
* [http://www.analyticsvidhya.com/blog/2019/06/understanding-transformers-nlp-state-of-the-art-models/ How do Transformers Work in NLP? A Guide to the Latest State-of-the-Art Models | Prateek Joshi - Analytics Vidhya] | * [http://www.analyticsvidhya.com/blog/2019/06/understanding-transformers-nlp-state-of-the-art-models/ How do Transformers Work in NLP? A Guide to the Latest State-of-the-Art Models | Prateek Joshi - Analytics Vidhya] | ||
* [https://jalammar.github.io/illustrated-gpt2/ Illustrated GPT-2 | Jay Alammmar] | * [https://jalammar.github.io/illustrated-gpt2/ Illustrated GPT-2 | Jay Alammmar] | ||
+ | * [https://medium.com/@lordmoma/the-transformer-model-revolutionizing-natural-language-processing-a16be54ddb1e The Transformer Model: Revolutionizing Natural Language Processing | David Lee - Medium] | ||
<b>Transformers</b> are [[Neural Network]] architectures using self-[[attention]] to understand [[context]] and long-term dependencies in language, used in many [[Natural Language Processing (NLP)]] applications such as chatbots and [[Sentiment Analysis]] tools. Transformer Model uniquely have [[attention]] such that every output element is connected to every input element. The weightings between them are calculated dynamically, effectively. [http://venturebeat.com/2019/10/24/google-achieves-state-of-the-art-nlp-performance-with-an-enormous-language-model-and-data-set/ | Kyle Wiggers] The dominant sequence transduction models are based on complex recurrent or convolutional neural networks in an [[Autoencoder (AE) / Encoder-Decoder]] configuration. The best performing models also connect the encoder and decoder through an attention mechanism. We propose a new simple network architecture, the Transformer, based solely on attention mechanisms, dispensing with recurrence and convolutions entirely. Experiments on two machine translation tasks show these models to be superior in quality while being more parallelizable and requiring significantly less time to train. [http://arxiv.org/abs/1706.03762 Attention Is All You Need | A. Vaswani, N. Shazeer, N. Parmar, J. Uszkoreit, L. Jones, A.N. Gomez, L. Kaiser, and I. Polosukhin] | <b>Transformers</b> are [[Neural Network]] architectures using self-[[attention]] to understand [[context]] and long-term dependencies in language, used in many [[Natural Language Processing (NLP)]] applications such as chatbots and [[Sentiment Analysis]] tools. Transformer Model uniquely have [[attention]] such that every output element is connected to every input element. The weightings between them are calculated dynamically, effectively. [http://venturebeat.com/2019/10/24/google-achieves-state-of-the-art-nlp-performance-with-an-enormous-language-model-and-data-set/ | Kyle Wiggers] The dominant sequence transduction models are based on complex recurrent or convolutional neural networks in an [[Autoencoder (AE) / Encoder-Decoder]] configuration. The best performing models also connect the encoder and decoder through an attention mechanism. We propose a new simple network architecture, the Transformer, based solely on attention mechanisms, dispensing with recurrence and convolutions entirely. Experiments on two machine translation tasks show these models to be superior in quality while being more parallelizable and requiring significantly less time to train. [http://arxiv.org/abs/1706.03762 Attention Is All You Need | A. Vaswani, N. Shazeer, N. Parmar, J. Uszkoreit, L. Jones, A.N. Gomez, L. Kaiser, and I. Polosukhin] | ||
+ | |||
+ | |||
+ | <hr><b><i>The transformer model in artificial intelligence, specifically in the realm of natural language processing, can be likened to a complex system of interconnected mini neural networks. Each of these mini networks is assigned to a token, which represents a piece of information or a word in a sentence. </b> In each mini neural network, there is a hierarchy of layers that perform computations. The main pathway, which can also be referred to as the 'residual stream' or 'main artery,' is where the information primarily flows. Occasionally, there are branches or 'offshoots' from this main pathway where additional computations are performed to enhance the understanding of the token. The results of these computations are then merged back into the main artery. The transformer model is hence a collection of these mini neural networks, each with a certain number of these branch-and-merge pathways. Smaller models may have around 12 to 24 of these, while larger models can have up to 100. Imagine a sentence, where each word or 'token' has its own dedicated mini transformer. These mini transformers continuously refine their understanding of their assigned tokens through iterative computations. What makes the transformer model unique and powerful is its ability to allow these mini transformers to exchange information with each other. Each mini transformer broadcasts information about what data they hold and what data they are looking for. Based on this, they fetch relevant information from other mini transformers and merge this new data into their own main artery. This process of information exchange and iterative computation is repeated cycle after cycle, refining the understanding of each token. After multiple cycles, the information reaches the bottom of the transformer stack. This information is then processed through a function called the [[Softmax]], which provides the final output in the form of probability distribution. In summary, the transformer model is an iterative information processing system. It processes each token independently while also allowing for information exchange between different tokens. This makes it a powerful tool for understanding the context and nuances in language. </i><hr> | ||
+ | |||
Competitive programming, conversational question answering, combinatorial optimization issues, and graph learning tasks all incorporate transformers as key components. Transformers models are used in competitive programming to produce solutions from textual descriptions. The well-known chatbot ChatGPT, which is a GPT-based model and a well-liked conversational question-answering model, is the best example of a transformer model. Transformers have also been used to resolve combinatorial optimization issues like the Travelling Salesman Problem, and they have been successful in graph learning tasks, especially when it comes to predicting the characteristics of molecules. Transformer models have shown great versatility in modalities, such as images, audio, video, and undirected [[Graph]]s. <i>[[Graph|Click here for directed graphs]]</i>. | Competitive programming, conversational question answering, combinatorial optimization issues, and graph learning tasks all incorporate transformers as key components. Transformers models are used in competitive programming to produce solutions from textual descriptions. The well-known chatbot ChatGPT, which is a GPT-based model and a well-liked conversational question-answering model, is the best example of a transformer model. Transformers have also been used to resolve combinatorial optimization issues like the Travelling Salesman Problem, and they have been successful in graph learning tasks, especially when it comes to predicting the characteristics of molecules. Transformer models have shown great versatility in modalities, such as images, audio, video, and undirected [[Graph]]s. <i>[[Graph|Click here for directed graphs]]</i>. | ||
The Transformer is a deep machine learning model introduced in 2017, used primarily in the field of natural language processing (NLP). Like [[Recurrent Neural Network (RNN)]], Transformers are designed to handle ordered sequences of data, such as natural language, for various tasks such as machine translation and text summarization. However, unlike RNNs, Transformers do not require that the sequence be processed in order. So, if the data in question is natural language, the Transformer does not need to process the beginning of a sentence before it processes the end. Due to this feature, the Transformer allows for much more parallelization than RNNs during training. Since their introduction, Transformers have become the basic building block of most state-of-the-art architectures in [[Natural Language Processing (NLP)]], replacing gated recurrent neural network models such as the [[Long Short-Term Memory (LSTM)]] in many cases. Since the Transformer architecture facilitates more parallelization during training computations, it has enabled training on much more data than was possible before it was introduced. This led to the development of pretrained systems such as [[Bidirectional Encoder Representations from Transformers (BERT)]] and [[Generative Pre-trained Transformer (GPT)]]-2, which have been trained with huge amounts of general language data prior to being released, and can then be fine-tune trained to specific language tasks.[http://en.wikipedia.org/wiki/Transformer_(machine_learning_model) Wikipedia] | The Transformer is a deep machine learning model introduced in 2017, used primarily in the field of natural language processing (NLP). Like [[Recurrent Neural Network (RNN)]], Transformers are designed to handle ordered sequences of data, such as natural language, for various tasks such as machine translation and text summarization. However, unlike RNNs, Transformers do not require that the sequence be processed in order. So, if the data in question is natural language, the Transformer does not need to process the beginning of a sentence before it processes the end. Due to this feature, the Transformer allows for much more parallelization than RNNs during training. Since their introduction, Transformers have become the basic building block of most state-of-the-art architectures in [[Natural Language Processing (NLP)]], replacing gated recurrent neural network models such as the [[Long Short-Term Memory (LSTM)]] in many cases. Since the Transformer architecture facilitates more parallelization during training computations, it has enabled training on much more data than was possible before it was introduced. This led to the development of pretrained systems such as [[Bidirectional Encoder Representations from Transformers (BERT)]] and [[Generative Pre-trained Transformer (GPT)]]-2, which have been trained with huge amounts of general language data prior to being released, and can then be fine-tune trained to specific language tasks.[http://en.wikipedia.org/wiki/Transformer_(machine_learning_model) Wikipedia] | ||
+ | |||
<youtube>4Bdc55j80l8</youtube> | <youtube>4Bdc55j80l8</youtube> | ||
+ | <youtube>kWLed8o5M2Y</youtube> | ||
+ | <youtube>acxqoltilME</youtube> | ||
<youtube>zxQyTK8quyY</youtube> | <youtube>zxQyTK8quyY</youtube> | ||
Line 71: | Line 82: | ||
* [https://huggingface.co/learn/nlp-course/chapter1/1 Welcome to the Course! | HuggingFace] | * [https://huggingface.co/learn/nlp-course/chapter1/1 Welcome to the Course! | HuggingFace] | ||
− | * [Large Language Model (LLM)#Token| | + | * [[Large Language Model (LLM)#Token|Tokens]] |
* [[Data Science]] ... [[Data Governance|Governance]] ... [[Data Preprocessing|Preprocessing]] ... [[Feature Exploration/Learning|Exploration]] ... [[Data Interoperability|Interoperability]] ... [[Algorithm Administration#Master Data Management (MDM)|Master Data Management (MDM)]] ... [[Bias and Variances]] ... [[Benchmarks]] ... [[Datasets]] | * [[Data Science]] ... [[Data Governance|Governance]] ... [[Data Preprocessing|Preprocessing]] ... [[Feature Exploration/Learning|Exploration]] ... [[Data Interoperability|Interoperability]] ... [[Algorithm Administration#Master Data Management (MDM)|Master Data Management (MDM)]] ... [[Bias and Variances]] ... [[Benchmarks]] ... [[Datasets]] | ||
Latest revision as of 18:02, 24 March 2024
YouTube ... Quora ...Google search ...Google News ...Bing News
- Large Language Model (LLM) ... Multimodal ... Foundation Models (FM) ... Generative Pre-trained ... Transformer ... GPT-4 ... GPT-5 ... Attention ... GAN ... BERT
- State Space Model (SSM) ... Mamba ... Sequence to Sequence (Seq2Seq) ... Recurrent Neural Network (RNN) ... Convolutional Neural Network (CNN)
- Natural Language Processing (NLP) ... Generation (NLG) ... Classification (NLC) ... Understanding (NLU) ... Translation ... Summarization ... Sentiment ... Tools
- Agents ... Robotic Process Automation ... Assistants ... Personal Companions ... Productivity ... Email ... Negotiation ... LangChain
- Excel ... Documents ... Database; Vector & Relational ... Graph ... LlamaIndex
- Artificial Intelligence (AI) ... Generative AI ... Machine Learning (ML) ... Deep Learning ... Neural Network ... Reinforcement ... Learning Techniques
- Conversational AI ... ChatGPT | OpenAI ... Bing/Copilot | Microsoft ... Gemini | Google ... Claude | Anthropic ... Perplexity ... You ... phind ... Ernie | Baidu
- Sequence to Sequence (Seq2Seq)
- Recurrent Neural Network (RNN)
- Long Short-Term Memory (LSTM)
- Autoencoder (AE) / Encoder-Decoder
- T5 (Text-To-Text Transfer Transformer) models
- Memory Networks
- Google Transformer-XL ...T5-XXL ...Google trained a trillion-parameter AI language model | Kyle Wiggers - VB
- The Illustrated Transformer | Jay Alammar
- Transformers Explained Visually (Part 1): Overview of Functionality | Ketan Doshi - Towards Data Science - Medium ... A Gentle Guide to Transformers, how they are used for Natural Language Processing (NLP), and why they are better than Recurrent Neural Network (RNN)s, in Plain English. How Attention helps improve performance. ... Part 2
- What is a Transformer? | Maxime Allard - Medium
- Transformers provides state-of-the-art general-purpose architectures (Bidirectional Encoder Representations from Transformers (BERT), Generative Pre-trained Transformer-2 (GPT-2), RoBERTa, XLM, DistilBert, XLNet...) for Natural Language Understanding (NLU) and Natural Language Generation (NLG) with over 32+ pretrained models in 100+ languages and deep interoperability between TensorFlow 2.0 and PyTorch. | GitHub
- How do Transformers Work in NLP? A Guide to the Latest State-of-the-Art Models | Prateek Joshi - Analytics Vidhya
- Illustrated GPT-2 | Jay Alammmar
- The Transformer Model: Revolutionizing Natural Language Processing | David Lee - Medium
Transformers are Neural Network architectures using self-attention to understand context and long-term dependencies in language, used in many Natural Language Processing (NLP) applications such as chatbots and Sentiment Analysis tools. Transformer Model uniquely have attention such that every output element is connected to every input element. The weightings between them are calculated dynamically, effectively. | Kyle Wiggers The dominant sequence transduction models are based on complex recurrent or convolutional neural networks in an Autoencoder (AE) / Encoder-Decoder configuration. The best performing models also connect the encoder and decoder through an attention mechanism. We propose a new simple network architecture, the Transformer, based solely on attention mechanisms, dispensing with recurrence and convolutions entirely. Experiments on two machine translation tasks show these models to be superior in quality while being more parallelizable and requiring significantly less time to train. Attention Is All You Need | A. Vaswani, N. Shazeer, N. Parmar, J. Uszkoreit, L. Jones, A.N. Gomez, L. Kaiser, and I. Polosukhin
The transformer model in artificial intelligence, specifically in the realm of natural language processing, can be likened to a complex system of interconnected mini neural networks. Each of these mini networks is assigned to a token, which represents a piece of information or a word in a sentence. In each mini neural network, there is a hierarchy of layers that perform computations. The main pathway, which can also be referred to as the 'residual stream' or 'main artery,' is where the information primarily flows. Occasionally, there are branches or 'offshoots' from this main pathway where additional computations are performed to enhance the understanding of the token. The results of these computations are then merged back into the main artery. The transformer model is hence a collection of these mini neural networks, each with a certain number of these branch-and-merge pathways. Smaller models may have around 12 to 24 of these, while larger models can have up to 100. Imagine a sentence, where each word or 'token' has its own dedicated mini transformer. These mini transformers continuously refine their understanding of their assigned tokens through iterative computations. What makes the transformer model unique and powerful is its ability to allow these mini transformers to exchange information with each other. Each mini transformer broadcasts information about what data they hold and what data they are looking for. Based on this, they fetch relevant information from other mini transformers and merge this new data into their own main artery. This process of information exchange and iterative computation is repeated cycle after cycle, refining the understanding of each token. After multiple cycles, the information reaches the bottom of the transformer stack. This information is then processed through a function called the Softmax, which provides the final output in the form of probability distribution. In summary, the transformer model is an iterative information processing system. It processes each token independently while also allowing for information exchange between different tokens. This makes it a powerful tool for understanding the context and nuances in language.
Competitive programming, conversational question answering, combinatorial optimization issues, and graph learning tasks all incorporate transformers as key components. Transformers models are used in competitive programming to produce solutions from textual descriptions. The well-known chatbot ChatGPT, which is a GPT-based model and a well-liked conversational question-answering model, is the best example of a transformer model. Transformers have also been used to resolve combinatorial optimization issues like the Travelling Salesman Problem, and they have been successful in graph learning tasks, especially when it comes to predicting the characteristics of molecules. Transformer models have shown great versatility in modalities, such as images, audio, video, and undirected Graphs. Click here for directed graphs.
The Transformer is a deep machine learning model introduced in 2017, used primarily in the field of natural language processing (NLP). Like Recurrent Neural Network (RNN), Transformers are designed to handle ordered sequences of data, such as natural language, for various tasks such as machine translation and text summarization. However, unlike RNNs, Transformers do not require that the sequence be processed in order. So, if the data in question is natural language, the Transformer does not need to process the beginning of a sentence before it processes the end. Due to this feature, the Transformer allows for much more parallelization than RNNs during training. Since their introduction, Transformers have become the basic building block of most state-of-the-art architectures in Natural Language Processing (NLP), replacing gated recurrent neural network models such as the Long Short-Term Memory (LSTM) in many cases. Since the Transformer architecture facilitates more parallelization during training computations, it has enabled training on much more data than was possible before it was introduced. This led to the development of pretrained systems such as Bidirectional Encoder Representations from Transformers (BERT) and Generative Pre-trained Transformer (GPT)-2, which have been trained with huge amounts of general language data prior to being released, and can then be fine-tune trained to specific language tasks.Wikipedia
Tensor2Tensor (T2T) | Google Brain
- Tensor2Tensor Transformers: New Deep Models for NLP | Łukasz Kaiser
- Tensor2Tensor | GitHub
- Tensor2Tensor Library | GitHub
- # Welcome to the Tensor2Tensor Colab
Tensor2Tensor, or T2T for short, is a library of deep learning models and datasets designed to make deep learning more accessible and [accelerate ML research](https://research.googleblog.com/2017/06/accelerating-deep-learning-research.html). T2T is actively used and maintained by researchers and engineers within the [Google Brain team](https://research.google.com/teams/brain/) and a community of users. This colab shows you some datasets we have in T2T, how to download and use them, some models we have, how to download pre-trained models and use them, and how to create and train your own models. | Jay Alammar]
Multi-head scaled dot-product attention mechanism. (Image source: Fig 2 in Vaswani, et al., 2017)
Transformers Course | HuggingFace
- Welcome to the Course! | HuggingFace
- Tokens
- Data Science ... Governance ... Preprocessing ... Exploration ... Interoperability ... Master Data Management (MDM) ... Bias and Variances ... Benchmarks ... Datasets
This course will teach you about natural language processing (NLP) using libraries from the Hugging Face ecosystem — 🤗 Transformers, 🤗 Datasets, 🤗 Tokenizers, and 🤗 Accelerate — as well as 🤗 the Hugging Face Hub.
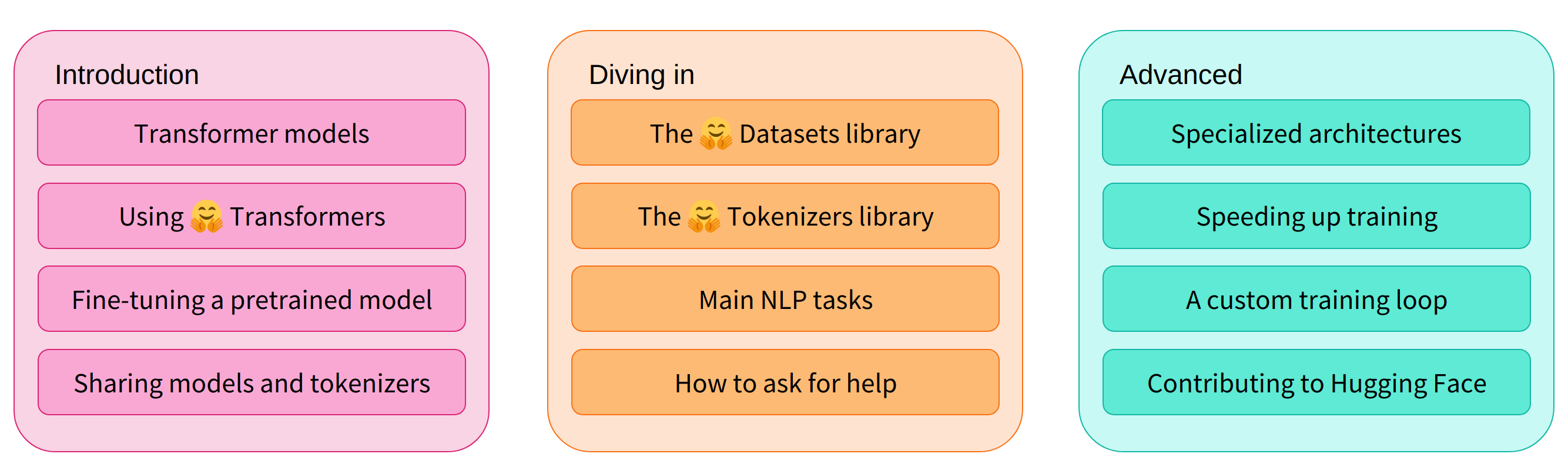
'https://elastic-ai.com/wp-content/uploads/2021/06/hfc_summary.png'
- Chapters 1 to 4 provide an introduction to the main concepts of the 🤗 Transformers library. By the end of this part of the course, you will be familiar with how Transformer models work and will know how to use a model from the Hugging Face Hub, fine-tune it on a dataset, and share your results on the Hub!
- Chapters 5 to 8 teach the basics of 🤗 Datasets and 🤗 Tokenizers before diving into classic NLP tasks. By the end of this part, you will be able to tackle the most common NLP problems by yourself.
- Chapters 9 to 12 go beyond NLP, and explore how Transformer models can be used to tackle tasks in speech processing and computer vision. Along the way, you’ll learn how to build and share demos of your models, and optimize them for production environments. By the end of this part, you will be ready to apply 🤗 Transformers to (almost) any machine learning problem!
This course:
- Requires a good knowledge of Python
- Is better taken after an introductory deep learning course, such as fast.ai’s Practical Deep Learning for Coders or one of the programs developed by DeepLearning.AI
- Does not expect prior PyTorch or TensorFlow knowledge, though some familiarity with either of those will help
After you’ve completed this course, we recommend checking out DeepLearning.AI’s Natural Language Processing Specialization, which covers a wide range of traditional NLP models like naive Bayes and Long Short-Term Memory (LSTM)s that are well worth knowing about!