Difference between revisions of "Reinforcement Learning (RL)"
m (→How does it work?) |
m |
||
(18 intermediate revisions by the same user not shown) | |||
Line 2: | Line 2: | ||
|title=PRIMO.ai | |title=PRIMO.ai | ||
|titlemode=append | |titlemode=append | ||
− | |keywords=artificial, intelligence, machine, learning, models | + | |keywords=ChatGPT, artificial, intelligence, machine, learning, GPT-4, GPT-5, NLP, NLG, NLC, NLU, models, data, singularity, moonshot, Sentience, AGI, Emergence, Moonshot, Explainable, TensorFlow, Google, Nvidia, Microsoft, Azure, Amazon, AWS, Hugging Face, OpenAI, Tensorflow, OpenAI, Google, Nvidia, Microsoft, Azure, Amazon, AWS, Meta, LLM, metaverse, assistants, agents, digital twin, IoT, Transhumanism, Immersive Reality, Generative AI, Conversational AI, Perplexity, Bing, You, Bard, Ernie, prompt Engineering LangChain, Video/Image, Vision, End-to-End Speech, Synthesize Speech, Speech Recognition, Stanford, MIT |description=Helpful resources for your journey with artificial intelligence; videos, articles, techniques, courses, profiles, and tools |
− | |description=Helpful resources for your journey with artificial intelligence; videos, articles, techniques, courses, profiles, and tools | + | |
+ | <!-- Google tag (gtag.js) --> | ||
+ | <script async src="https://www.googletagmanager.com/gtag/js?id=G-4GCWLBVJ7T"></script> | ||
+ | <script> | ||
+ | window.dataLayer = window.dataLayer || []; | ||
+ | function gtag(){dataLayer.push(arguments);} | ||
+ | gtag('js', new Date()); | ||
+ | |||
+ | gtag('config', 'G-4GCWLBVJ7T'); | ||
+ | </script> | ||
}} | }} | ||
[https://www.youtube.com/results?search_query=ai+Reinforcement+Learning YouTube] | [https://www.youtube.com/results?search_query=ai+Reinforcement+Learning YouTube] | ||
Line 11: | Line 20: | ||
[https://www.bing.com/news/search?q=ai+Reinforcement+Learning&qft=interval%3d%228%22 ...Bing News] | [https://www.bing.com/news/search?q=ai+Reinforcement+Learning&qft=interval%3d%228%22 ...Bing News] | ||
− | * [[What is Artificial Intelligence (AI)? | Artificial Intelligence (AI)]] ... [[Machine Learning (ML)]] ... [[Deep Learning]] ... [[Neural Network]] ... [[Reinforcement Learning (RL)|Reinforcement]] ... [[Learning Techniques]] | + | * [[What is Artificial Intelligence (AI)? | Artificial Intelligence (AI)]] ... [[Generative AI]] ... [[Machine Learning (ML)]] ... [[Deep Learning]] ... [[Neural Network]] ... [[Reinforcement Learning (RL)|Reinforcement]] ... [[Learning Techniques]] |
− | + | * [[Reinforcement Learning - Games, Self-driving Vehicles, Drones, Robotics, Management, Finance]] | |
− | + | * [[Conversational AI]] ... [[ChatGPT]] | [[OpenAI]] ... [[Bing/Copilot]] | [[Microsoft]] ... [[Gemini]] | [[Google]] ... [[Claude]] | [[Anthropic]] ... [[Perplexity]] ... [[You]] ... [[phind]] ... [[Grok]] | [https://x.ai/ xAI] ... [[Groq]] ... [[Ernie]] | [[Baidu]] ... [[DeepSeek]] | |
− | + | * [[Agents]] ... [[Robotic Process Automation (RPA)|Robotic Process Automation]] ... [[Assistants]] ... [[Personal Companions]] ... [[Personal Productivity|Productivity]] ... [[Email]] ... [[Negotiation]] ... [[LangChain]] | |
− | |||
− | * [[Assistants]] | ||
* [[Inverse Reinforcement Learning (IRL)]] | * [[Inverse Reinforcement Learning (IRL)]] | ||
− | * [[Game Theory]] | + | * [[Gaming]] ... [[Game-Based Learning (GBL)]] ... [[Games - Security|Security]] ... [[Game Development with Generative AI|Generative AI]] ... [[Metaverse#Games - Metaverse|Games - Metaverse]] ... [[Games - Quantum Theme|Quantum]] ... [[Game Theory]] ... [[Game Design | Design]] |
+ | * [[Robotics]] ... [[Transportation (Autonomous Vehicles)|Vehicles]] ... [[Autonomous Drones|Drones]] ... [[3D Model]] ... [[Point Cloud]] | ||
+ | * [[Simulation]] ... [[Simulated Environment Learning]] ... [[World Models]] ... [[Minecraft]]: [[Minecraft#Voyager|Voyager]] | ||
* [[Policy]] ... [[Policy vs Plan]] ... [[Constitutional AI]] ... [[Trust Region Policy Optimization (TRPO)]] ... [[Policy Gradient (PG)]] ... [[Proximal Policy Optimization (PPO)]] | * [[Policy]] ... [[Policy vs Plan]] ... [[Constitutional AI]] ... [[Trust Region Policy Optimization (TRPO)]] ... [[Policy Gradient (PG)]] ... [[Proximal Policy Optimization (PPO)]] | ||
− | |||
* [[Multi-Task Learning (MTL)]] ... [[SMART - Multi-Task Deep Neural Networks (MT-DNN)]] | * [[Multi-Task Learning (MTL)]] ... [[SMART - Multi-Task Deep Neural Networks (MT-DNN)]] | ||
* [[AdaNet]] | * [[AdaNet]] | ||
* [[Loop#Feedback Loop - The AI Economist|Feedback Loop - The AI Economist]] | * [[Loop#Feedback Loop - The AI Economist|Feedback Loop - The AI Economist]] | ||
* [[Dopamine]] Google DeepMind | * [[Dopamine]] Google DeepMind | ||
− | + | * [[Math for Intelligence]] ... [[Finding Paul Revere]] ... [[Social Network Analysis (SNA)]] ... [[Dot Product]] ... [[Kernel Trick]] | |
− | * [[Inside Out - Curious Optimistic Reasoning]] | + | * [[Artificial General Intelligence (AGI) to Singularity]] ... [[Inside Out - Curious Optimistic Reasoning| Curious Reasoning]] ... [[Emergence]] ... [[Moonshots]] ... [[Explainable / Interpretable AI|Explainable AI]] ... [[Algorithm Administration#Automated Learning|Automated Learning]] |
* [[World Models]] | * [[World Models]] | ||
* [[Google DeepMind AlphaGo Zero]] | * [[Google DeepMind AlphaGo Zero]] | ||
Line 39: | Line 47: | ||
* [https://learn.microsoft.com/en-us/azure/architecture/solution-ideas/articles/machine-teaching Use subject matter expertise in machine teaching and reinforcement learning | Microsoft] | * [https://learn.microsoft.com/en-us/azure/architecture/solution-ideas/articles/machine-teaching Use subject matter expertise in machine teaching and reinforcement learning | Microsoft] | ||
* [https://www.nature.com/articles/s41586-023-06004-9 Faster sorting algorithms discovered using deep reinforcement learning | D. Mankowitz, A. Michi, A. Zhernov, M. Gelmi, M. Selvi, C. Paduraru, E. Leurent, S. Iqbal, J. Lespiau, A. Ahern, T. Köppe, K. Millikin, S. Gaffney, S. Elster, J. Broshear, C. Gamble, K. Milan, R. Tung, M. Hwang, T. Cemgil, M. Barekatain, Y. Li, A. Mandhane, T. Hubert, D. Silver - Nature] ... Deep reinforcement learning has been used to improve computer code by treating the task as a game — with no special knowledge needed on the part of the player. The result has already worked its way into countless programs. | * [https://www.nature.com/articles/s41586-023-06004-9 Faster sorting algorithms discovered using deep reinforcement learning | D. Mankowitz, A. Michi, A. Zhernov, M. Gelmi, M. Selvi, C. Paduraru, E. Leurent, S. Iqbal, J. Lespiau, A. Ahern, T. Köppe, K. Millikin, S. Gaffney, S. Elster, J. Broshear, C. Gamble, K. Milan, R. Tung, M. Hwang, T. Cemgil, M. Barekatain, Y. Li, A. Mandhane, T. Hubert, D. Silver - Nature] ... Deep reinforcement learning has been used to improve computer code by treating the task as a game — with no special knowledge needed on the part of the player. The result has already worked its way into countless programs. | ||
+ | * [https://towardsdatascience.com/develop-your-first-ai-agent-deep-q-learning-375876ee2472 Develop Your First AI Agent: Deep Q-Learning | Heston Vaughan - Towards Data Science] | ||
<b>Reinforcement Learning (RL)</b> A technique that teaches an AI model to find the best result through trial and error and receiving rewards or punishments based on its results, often enhanced by human feedback for games and complex tasks. | <b>Reinforcement Learning (RL)</b> A technique that teaches an AI model to find the best result through trial and error and receiving rewards or punishments based on its results, often enhanced by human feedback for games and complex tasks. | ||
Line 47: | Line 56: | ||
[http://venturebeat.com/2021/06/09/deepmind-says-reinforcement-learning-is-enough-to-reach-general-ai/ Some scientists believe that assembling multiple narrow AI modules will produce higher intelligent systems.]</i> | [http://venturebeat.com/2021/06/09/deepmind-says-reinforcement-learning-is-enough-to-reach-general-ai/ Some scientists believe that assembling multiple narrow AI modules will produce higher intelligent systems.]</i> | ||
− | </center><hr> | + | </center><hr> |
− | http://slideplayer.com/24/7469154/big_thumb.jpg | + | <center>http://slideplayer.com/24/7469154/big_thumb.jpg</center> |
Line 55: | Line 64: | ||
This is a bit similar to the traditional type of data analysis; the algorithm discovers through trial and error and decides which action results in greater rewards. Three major components can be identified in reinforcement learning functionality: the [[Agents|agent]], the environment, and the actions. The [[Agents|agent]] is the learner or decision-maker, the environment includes everything that the [[Agents|agent]] interacts with, and the actions are what the [[Agents|agent]] can do. Reinforcement learning occurs when the [[Agents|agent]] chooses actions that maximize the expected reward over a given time. This is best achieved when the [[Agents|agent]] has a good policy to follow. [http://www.simplilearn.com/what-is-machine-learning-and-why-it-matters-article Machine Learning: What it is and Why it Matters | Priyadharshini @ simplilearn] | This is a bit similar to the traditional type of data analysis; the algorithm discovers through trial and error and decides which action results in greater rewards. Three major components can be identified in reinforcement learning functionality: the [[Agents|agent]], the environment, and the actions. The [[Agents|agent]] is the learner or decision-maker, the environment includes everything that the [[Agents|agent]] interacts with, and the actions are what the [[Agents|agent]] can do. Reinforcement learning occurs when the [[Agents|agent]] chooses actions that maximize the expected reward over a given time. This is best achieved when the [[Agents|agent]] has a good policy to follow. [http://www.simplilearn.com/what-is-machine-learning-and-why-it-matters-article Machine Learning: What it is and Why it Matters | Priyadharshini @ simplilearn] | ||
− | + | Control-based: When running a Reinforcement Learning (RL) policy in the real world, such as controlling a physical robot on visual inputs, it is non-trivial to properly track states, obtain reward signals or determine whether a goal is achieved for real. The visual data has a lot of noise that is irrelevant to the true state and thus the equivalence of states cannot be inferred from pixel-level comparison. Self-supervised representation learning has shown great potential in learning useful state [[embedding]] that can be used directly as input to a control policy. | |
− | |||
− | Control-based: When running a Reinforcement Learning (RL) policy in the real world, such as controlling a physical robot on visual inputs, it is non-trivial to properly track states, obtain reward signals or determine whether a goal is achieved for real. The visual data has a lot of noise that is irrelevant to the true state and thus the equivalence of states cannot be inferred from pixel-level comparison. Self-supervised representation learning has shown great potential in learning useful state embedding that can be used directly as input to a control policy. | ||
− | <center><img src="https://adatis.co.uk/wp-content/uploads/Reinforcement-Learning-SMALL.gif" width=" | + | <center><img src="https://adatis.co.uk/wp-content/uploads/Reinforcement-Learning-SMALL.gif" width="800"></center> |
<youtube>e3Jy2vShroE</youtube> | <youtube>e3Jy2vShroE</youtube> |
Latest revision as of 05:58, 30 January 2025
YouTube ... Quora ...Google search ...Google News ...Bing News
- Artificial Intelligence (AI) ... Generative AI ... Machine Learning (ML) ... Deep Learning ... Neural Network ... Reinforcement ... Learning Techniques
- Reinforcement Learning - Games, Self-driving Vehicles, Drones, Robotics, Management, Finance
- Conversational AI ... ChatGPT | OpenAI ... Bing/Copilot | Microsoft ... Gemini | Google ... Claude | Anthropic ... Perplexity ... You ... phind ... Grok | xAI ... Groq ... Ernie | Baidu ... DeepSeek
- Agents ... Robotic Process Automation ... Assistants ... Personal Companions ... Productivity ... Email ... Negotiation ... LangChain
- Inverse Reinforcement Learning (IRL)
- Gaming ... Game-Based Learning (GBL) ... Security ... Generative AI ... Games - Metaverse ... Quantum ... Game Theory ... Design
- Robotics ... Vehicles ... Drones ... 3D Model ... Point Cloud
- Simulation ... Simulated Environment Learning ... World Models ... Minecraft: Voyager
- Policy ... Policy vs Plan ... Constitutional AI ... Trust Region Policy Optimization (TRPO) ... Policy Gradient (PG) ... Proximal Policy Optimization (PPO)
- Multi-Task Learning (MTL) ... SMART - Multi-Task Deep Neural Networks (MT-DNN)
- AdaNet
- Feedback Loop - The AI Economist
- Dopamine Google DeepMind
- Math for Intelligence ... Finding Paul Revere ... Social Network Analysis (SNA) ... Dot Product ... Kernel Trick
- Artificial General Intelligence (AGI) to Singularity ... Curious Reasoning ... Emergence ... Moonshots ... Explainable AI ... Automated Learning
- World Models
- Google DeepMind AlphaGo Zero
- Neural Architecture Search (NAS) with Reinforcement Learning | Barret Zoph & Quoc V. Le ...Wikipedia
- Beyond DQN/A3C: A Survey in Advanced Reinforcement Learning | Joyce Xu - Towards Data Science
- Google’s AI picks which machine learning models will produce the best results | Kyle Wiggers - VentureBeat off-policy classification,” or OPC, which evaluates the performance of AI-driven agents by treating evaluation as a classification problem
- Deep Reinforcement Learning Hands-On: Apply modern RL methods, with deep Q-networks, value iteration, policy gradients, TRPO, AlphaGo Zero and more | Maxim Lapan
- Reinforcement-Learning-Notebooks - A collection of Reinforcement Learning algorithms from Sutton and Barto's book and other research papers implemented in Python
- What is Reinforcement Learning? | Daniel Nelson - Unite.ai
- Reinforcement Learning (RL) | Wikipedia
- A Beginner's Guide to Deep Reinforcement Learning | Chris Nicholson - A.I. Wiki pathmind
- Use subject matter expertise in machine teaching and reinforcement learning | Microsoft
- Faster sorting algorithms discovered using deep reinforcement learning | D. Mankowitz, A. Michi, A. Zhernov, M. Gelmi, M. Selvi, C. Paduraru, E. Leurent, S. Iqbal, J. Lespiau, A. Ahern, T. Köppe, K. Millikin, S. Gaffney, S. Elster, J. Broshear, C. Gamble, K. Milan, R. Tung, M. Hwang, T. Cemgil, M. Barekatain, Y. Li, A. Mandhane, T. Hubert, D. Silver - Nature ... Deep reinforcement learning has been used to improve computer code by treating the task as a game — with no special knowledge needed on the part of the player. The result has already worked its way into countless programs.
- Develop Your First AI Agent: Deep Q-Learning | Heston Vaughan - Towards Data Science
Reinforcement Learning (RL) A technique that teaches an AI model to find the best result through trial and error and receiving rewards or punishments based on its results, often enhanced by human feedback for games and complex tasks.
DeepMind says reinforcement learning is enough to reach Artificial General Intelligence (AGI)
...
Some scientists believe that assembling multiple narrow AI modules will produce higher intelligent systems.
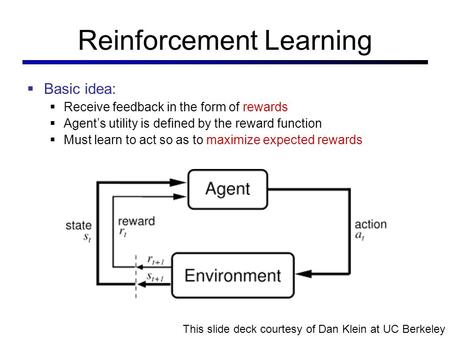
Contents
[hide]How does it work?
This is a bit similar to the traditional type of data analysis; the algorithm discovers through trial and error and decides which action results in greater rewards. Three major components can be identified in reinforcement learning functionality: the agent, the environment, and the actions. The agent is the learner or decision-maker, the environment includes everything that the agent interacts with, and the actions are what the agent can do. Reinforcement learning occurs when the agent chooses actions that maximize the expected reward over a given time. This is best achieved when the agent has a good policy to follow. Machine Learning: What it is and Why it Matters | Priyadharshini @ simplilearn
Control-based: When running a Reinforcement Learning (RL) policy in the real world, such as controlling a physical robot on visual inputs, it is non-trivial to properly track states, obtain reward signals or determine whether a goal is achieved for real. The visual data has a lot of noise that is irrelevant to the true state and thus the equivalence of states cannot be inferred from pixel-level comparison. Self-supervised representation learning has shown great potential in learning useful state embedding that can be used directly as input to a control policy.

Reinforcement Learning (RL) Algorithms
- Reinforcement Learning (RL) from Human Feedback (RLHF)
- In-context Reinforcement Learning
- Monte Carlo (MC) Method - Model Free Reinforcement Learning
- Markov Decision Process (MDP)
- State-Action-Reward-State-Action (SARSA)
- Q Learning
- Deep Reinforcement Learning (DRL) DeepRL
- Distributed Deep Reinforcement Learning (DDRL)
- Evolutionary Computation / Genetic Algorithms
- Actor Critic
- Hierarchical Reinforcement Learning (HRL)
- Apprenticeship Learning - Inverse Reinforcement Learning (IRL)
- Lifelong Learning
Q Learning Algorithm and Agent - Reinforcement Learning w/ Python Tutorial | Sentdex - Harrison
Reinforcement Learning | Phil Tabor
Reinforcement learning is an area of machine learning that involves taking right action to maximize reward in a particular situation. In this full tutorial course, you will get a solid foundation in reinforcement learning core topics. The course covers Q learning, State-Action-Reward-State-Action (SARSA), double Q learning, Deep Q Learning (DQN), and Policy Gradient (PG) methods. These algorithms are employed in a number of environments from the open AI gym, including space invaders, breakout, and others. The deep learning portion uses Tensorflow and PyTorch. The course begins with more modern algorithms, such as deep q learning and Policy Gradient (PG) methods, and demonstrates the power of reinforcement learning. Then the course teaches some of the fundamental concepts that power all reinforcement learning algorithms. These are illustrated by coding up some algorithms that predate deep learning, but are still foundational to the cutting edge. These are studied in some of the more traditional environments from the OpenAI Gym, like the cart pole problem.
⌨️ (00:00:00) Introduction
⌨️ (00:01:30) Intro to Deep Q Learning
⌨️ (00:08:56) How to Code Deep Q Learning in Tensorflow
⌨️ (00:52:03) Deep Q Learning with Pytorch Part 1: The Q Network
⌨️ (01:06:21) Deep Q Learning with Pytorch part 2: Coding the Agent
⌨️ (01:28:54) Deep Q Learning with Pytorch part 3
⌨️ (01:46:39) Intro to Policy Gradients 3: Coding the main loop
⌨️ (01:55:01) How to Beat Lunar Lander with Policy Gradients
⌨️ (02:21:32) How to Beat Space Invaders with Policy Gradients
⌨️ (02:34:41) How to Create Your Own Reinforcement Learning Environment Part 1
⌨️ (02:55:39) How to Create Your Own Reinforcement Learning Environment Part 2
⌨️ (03:08:20) Fundamentals of Reinforcement Learning
⌨️ (03:17:09) Markov Decision Processes
⌨️ (03:23:02) The Explore Exploit Dilemma
⌨️ (03:29:19) Reinforcement Learning in the Open AI Gym: SARSA
⌨️ (03:39:56) Reinforcement Learning in the Open AI Gym: Double Q Learning
⌨️ (03:54:07) Conclusion
Jump Start
Gridworld: How To Create Your Own Reinforcement Learning Environments