Difference between revisions of "Agents"
m (→Multi-Agents Reinforcement Learning (MARL)) |
m |
||
Line 599: | Line 599: | ||
</i></center><hr> | </i></center><hr> | ||
+ | |||
+ | = Multi-Agents Reinforcement Learning (MARL) = | ||
+ | * [https://arxiv.org/abs/1911.10635 Multi-Agent Reinforcement Learning: A Selective Overview of Theories and Algorithms | K. Zhang, Z. Yang, and T. Başar] | ||
+ | |||
+ | In a similar vein, multi-agent RL also addresses sequential decision-making problems, but | ||
+ | with more than one agent involved. In particular, both the evolution of the system state | ||
+ | and the reward received by each agent are influenced by the joint actions of all agents. | ||
+ | More intriguingly, each agent has its own long-term reward to optimize, which now becomes a function of the policies of all other agents. | ||
+ | |||
+ | * Markov/Stochastic Games | ||
+ | ** Cooperative Setting | ||
+ | ** Competitive Setting | ||
+ | ** Mixed Setting | ||
+ | * Extensive-Form Games | ||
+ | |||
+ | == Challenges == | ||
+ | Despite a general model with broad applications, MARL suffers from several challenges | ||
+ | in theoretical analysis, in addition to those that arise in single-agent RL. | ||
+ | |||
+ | # Non-Unique Learning Goals - Unlike single-agent RL, where the goal of the agent is to maximize the long-term return efficiently, the learning goals of MARL can be vague at times. ...Indeed, the goals that need to be considered in the analysis of MARL algorithms can be multi-dimensional ... is undoubtedly a reasonable solution concept in game theory, under the assumption that the agents are all rational, and are capable of perfectly reasoning and infinite mutual modeling of agents. However, with bounded rationality, the agents may only be able to perform finite mutual modeling | ||
+ | # Non-Stationarity - multiple agents usually learn concurrently, causing the environment faced by each individual agent to be non-stationary. In particular, the action taken by one agent affects the reward of other opponent agents, and the evolution of the state. As a result, the learning agent is required to account for how the other agents behave and adapt to the joint behavior accordingly. This invalidates the stationarity assumption for establishing the convergence of single-agent RL algorithms | ||
+ | # Scalability Issue - To handle non-stationarity, each individual agent may need to account for the joint action space, whose dimension increases exponentially with the number of agents. This is also referred to as the combinatorial nature of MARL | ||
+ | # Various Information Structures - Compared to the single-agent case, the information structure of MARL, namely, who knows what at the training and execution, is more involved. For example, in the framework of Markov games, it suffices to observe the instantaneous state st , in order for each agent to make decisions | ||
+ | |||
+ | <youtube>Yd6HNZnqjis</youtube> | ||
+ | <youtube>Gv-8X6tNPNw</youtube> | ||
+ | <youtube>nTPuL6iVuwU</youtube> | ||
+ | <youtube>p_n5fF8apiE</youtube> |
Revision as of 15:37, 1 March 2024
Youtube ... Quora ...Google search ...Google News ...Bing News
- Assistants ... Personal Companions ... Agents ... Negotiation ... LangChain
- Assistant: Reactive; task completion
- Personal Companion: Proactive and reactive; building a relationship with the user
- Agent: Proactive; performing tasks, making decisions on behalf of the user
- Development ... Notebooks ... AI Pair Programming ... Codeless, Generators, Drag n' Drop ... AIOps/MLOps ... AIaaS/MLaaS
- Immersive Reality ... Metaverse ... Digital Twin ... Internet of Things (IoT) ... Transhumanism
- Artificial General Intelligence (AGI) to Singularity ... Curious Reasoning ... Emergence ... Moonshots ... Explainable AI ... Automated Learning
- Python ... GenAI w/ Python ... JavaScript ... GenAI w/ JavaScript ... TensorFlow ... PyTorch
- Gaming ... Game-Based Learning (GBL) ... Security ... Generative AI ... Metaverse ... Quantum ... Game Theory
- Artificial Intelligence (AI) ... Generative AI ... Machine Learning (ML) ... Deep Learning ... Neural Network ... Reinforcement ... Learning Techniques
- Conversational AI ... ChatGPT | OpenAI ... Bing | Microsoft ... Bard | Google ... Claude | Anthropic ... Perplexity ... You ... Ernie | Baidu
- ChatGPT Integration
- Minecraft: Voyager ... an AI agent powered by a Large Language Model (LLM) that has been introduced to the world of Minecraft
- Large Language Model (LLM) ... Natural Language Processing (NLP) ...Generation ... Classification ... Understanding ... Translation ... Tools & Services
- Attention Mechanism ...Transformer ...Generative Pre-trained Transformer (GPT) ... GAN ... BERT
- Artificial Intelligence (AI) ... Machine Learning (ML) ... Deep Learning ... Neural Network ... Reinforcement ... Learning Techniques
- Policy ... Policy vs Plan ... Constitutional AI ... Trust Region Policy Optimization (TRPO) ... Policy Gradient (PG) ... Proximal Policy Optimization (PPO)
- Multi-Task Learning (MTL) ... SMART - Multi-Task Deep Neural Networks (MT-DNN)
- Deep Distributed Q Network Partial Observability
- Metaverse#AI TownAI Town ... a virtual town where AI characters live, chat and socialize.
- What Is A Virtual Agent & What Can It Do For Your Business? | Talkative
- Types of AI Agents | JavaTpoint ... Simple Reflex, Model-based reflex, Goal-based, Utility-based, Learning agents
- Key Research Advances in Building Task-Oriented Dialog Agents | Mariya Yao - TOPBOTS
- MineDojo ... framework built on the popular Minecraft game for embodied agent research
- Google Tells AI Agents to Behave Like 'Believable Humans' to Create 'Artificial Society' | Chloe Xiang - Vice ... The characters have developed specific routines, such as waking up, taking a shower, cooking breakfast, interacting with their families, then going to work every day... researchers input one paragraph per character into ChatGPT
- Amazon creates a new user-centric simulation platform to develop embodied AI agents | Ingrid Fadelli - TechExplore ... Arena is a new Embodied AI platform, for robotic task completion in simulated environments.
- Your AI Council ... assesses queries through various agents, offering insights from many perspectives.
The paradigm of AI copilots is undergoing a transformation, shifting from AI serving as a supportive assistant to humans, to humans taking on the role of AI copilots. This shift represents a significant change in how individuals interact with AI, enabling users to harness the power of AI to enhance decision-making and efficiency. This shift in the copilot paradigm signifies a move towards a more collaborative and empowering relationship between humans and AI, where humans actively engage with AI to augment their capabilities and productivity.
Contents
There are 3 stages; Beside, Inside, & Outside - Steven Bathiche
- AI will be beside what we are doing: AI is a valuable tool; a helper, a sidebar, an assistant that can aid us in many aspects of our lives. As a conversational AI, it can help us with research, writing, and presentations, breaking our mental blacks, managing our personal lives by scheduling appointments and managing our finances. By automating tasks such as data entry and email processing, AI frees up our time so that we can focus on more important tasks.
- AI will inside what we are doing: AI will become even more integrated into our daily lives, understanding our needs and preferences and anticipating our actions. It will be everywhere, acting as the scaffolding for our interactions with technology. Instead of waiting for us to make a keystroke or select an application, AI will take the lead, choosing the best app for us and coaching us through a vast array of possibilities.
- AI will be outside what we are doing: AI agents will act as intermediaries, pulling information from disparate data sources and interpreting the world for us. They will help us navigate and understand the context, acting as facilitators and eliminating the challenge of starting with a blank sheet.
An intelligent agent is anything which perceives its environment, takes actions autonomously in order to achieve goals, and may improve its performance with learning or acquiring knowledge. An agent has an "objective function" that encapsulates all the IA's goals. Such an agent is designed to create and execute whatever plan will, upon completion, maximize the expected value of the objective function.[2] For example, a reinforcement learning agent has a "reward function" that allows the programmers to shape the IA's desired behavior,[3] and an evolutionary algorithm's behavior is shaped by a "fitness function". - Wikipedia
What is an AI Agent?
- AI Tools and Autonomous Agents: Auto-GPT, BabyAGI, LangChain, AgentGPT, HeyGPT, and more | Anna Geller - Medium ... Summary of recent trends for AI end-users (rather than AI engineers or researchers)
LlamaIndex’s Agent
LlamaIndex's Agent is a large language model (LLM) chatbot developed by LlamaIndex, a company that specializes in alpaca and llama products and information. The agent is trained on a massive dataset of text and code, including LlamaIndex's own database of alpaca and llama information. This allows the agent to answer a wide range of questions about alpacas and llamas, including their biology, behavior, care, and products. The agent can also be used to generate creative text formats of text content, like poems, code, scripts, musical pieces, email, letters, etc. It can also be used to translate languages. LlamaIndex's Agent is a valuable resource for anyone who is interested in learning more about alpacas and llamas. It is also a useful tool for alpaca and llama breeders, farmers, and businesses. Here are some examples of how LlamaIndex's Agent can be used:
- Answer questions about alpacas and llamas: The agent can answer a wide range of questions about alpacas and llamas, including their biology, behavior, care, and products. For example, you could ask the agent "How long do alpacas live?" or "What is the difference between a llama and an alpaca?"
- Generate creative text formats: The agent can be used to generate creative text formats of text content, like poems, code, scripts, musical pieces, email, letters, etc. For example, you could ask the agent to write a poem about alpacas or a script for a video about llama care.
- Translate languages: The agent can also be used to translate languages. For example, you could ask the agent to translate a Spanish article about alpacas into English.
Langchain’s Agent
LangChain's Agent is a type of artificial intelligence (AI) that can be used to automate a variety of tasks. It is a language model that is trained on a massive dataset of text and code. This allows the agent to understand and respond to natural language, and to perform many kinds of tasks, including:
- Answering questions in a comprehensive and informative way
- Generating different creative text formats of text content
- Translating languages
- Automating tasks that would otherwise require human intervention
LangChain's Agent is still under development, but it has the potential to revolutionize the way we work and live. For example, it could be used to develop new types of customer service chatbots, to automate tasks in software development, and to create new forms of creative content. Here are some examples of how LangChain's Agent could be used in the real world:
- A customer service chatbot could use LangChain's Agent to answer customer questions in a more comprehensive and informative way than traditional chatbots.
- A software development team could use LangChain's Agent to automate tasks such as writing unit tests and generating documentation.
- A writer could use LangChain's Agent to generate ideas for new articles or stories, or to translate their work into other languages.
LangChain's Agent is a powerful tool that has the potential to be used in a wide variety of applications. As the technology continues to develop, we can expect to see even more innovative and exciting uses for LangChain's Agent in the future. In addition to the examples above, LangChain's Agent could also be used to:
- Develop new educational tools that can personalize instruction and provide feedback to students.
- Create new types of creative content, such as interactive stories and games.
- Develop new tools for scientific research, such as helping scientists to design experiments and analyze data.
- Automate tasks in a variety of industries, such as healthcare, finance, and manufacturing.
DeepMind's WebAgent
YouTube ... Quora ...Google search ...Google News ...Bing News
- A Real-World WebAgent with Planning, Long Context Understanding, and Program Synthesis | I. Gur, H. Furuta, A. Huang, M. Safdari, Y. Matsuo, D. Eck, & A. Faust
- Meet WebAgent: DeepMind’s New LLM that Follows Instructions and Complete Tasks on Websites Google | Jesus Rodriguez - Towards AI] ... The model combines language understanding and web navigation.
- Google DeepMind and the University of Tokyo Researchers Introduce WebAgent: An LLM-Driven Agent that can Complete the Tasks on Real Websites Following Natural Language Instructions | Aneesh Tickoo - MarketTechPost
Google DeepMind's WebAgent can autonomously navigate and interact with websites, following natural language instructions to complete tasks; capable of understanding and interacting with the vast and complex world of the internet.
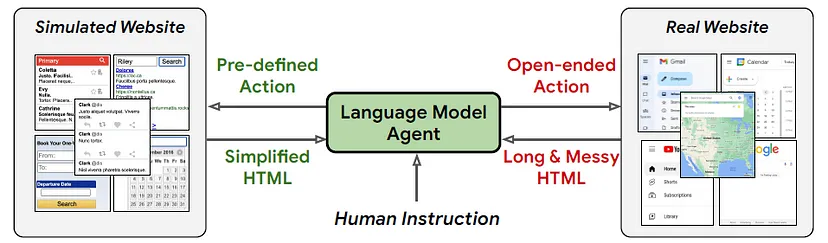
'https://miro.medium.com/v2/resize:fit:828/format:webp/1*sA904oL8A4DUQ6FQInNAbw.png'
Key Features of WebAgent:
- Planning and Long Context Understanding: WebAgent can break down complex instructions into smaller, manageable steps and analyze lengthy HTML documents to extract relevant information. This allows it to effectively plan its actions and adapt to the dynamic nature of websites.
- Large Language Model (LLM): WebAgent's design incorporates two distinct large language models, HTML-T5 and Flan-U-PaLM, each specialized for specific tasks. This modular approach allows for efficient processing and enhanced performance. HTML-T5: A domain-specific LLM trained on a massive corpus of HTML documents, specializing in understanding and summarizing website content. Flan-U-PaLM: A powerful LLM capable of generating executable Python code, enabling WebAgent to interact with websites directly.
- Program Synthesis: WebAgent employs program synthesis techniques to translate natural language instructions into executable code, allowing it to perform actions on websites. WebAgent can generate executable Python code based on its understanding of instructions and website structure. This enables it to directly interact with websites, filling forms, clicking buttons, and extracting data.
- Reinforcement Learning (RL): Reinforcement learning algorithms are used to train WebAgent, enabling it to learn from experience and improve its performance over time.
- Web Scraping Techniques: WebAgent utilizes web scraping techniques to extract relevant information from websites, such as text, images, and links.
- Natural Language Processing (NLP): NLP techniques are employed to analyze and understand natural language instructions, enabling WebAgent to follow complex commands.
- Machine Learning (ML): Machine learning algorithms are used to train and optimize WebAgent's various components, enhancing its ability to perform tasks accurately and efficiently.
Potential Applications:
- Automated Web Testing: WebAgent can be employed to automate website testing, identifying bugs, and ensuring functionality across different platforms and browsers.
- Web Scraping and Data Extraction: WebAgent's ability to understand and navigate websites makes it an ideal tool for extracting specific data from various sources, streamlining data collection processes.
- Personalized Web Assistance: WebAgent could serve as a personalized web assistant, helping users with tasks like booking appointments, making online purchases, or managing personal accounts.
Jarvis
YouTube ... Quora ...Google search ...Google News ...Bing News
Jarvis is a project from Microsoft that uses ChatGPT as the controller for a system where it can employ a variety of other models as needed to respond to your prompt. Microsoft Jarvis connects LLMs with ML community. Language serves as an interface for Large Language Model (LLM)s to connect numerous AI models for solving complicated AI tasks. Solving complicated AI tasks with different domains and modalities is a key step toward advanced artificial intelligence. While there are abundant AI models available for different domains and modalities, they cannot handle complicated AI tasks. Considering Large Language Model (LLM) have exhibited exceptional ability in language understanding, generation, interaction, and reasoning, we advocate that LLMs could act as a controller to manage existing AI models to solve complicated AI tasks and language could be a generic interface to empower this.
HuggingGPT is one instance of Jarvis, a web-based chatbot at Hugging Face, an online AI community which hosts thousands of open-source models.
When a user makes a request to the bot, Jarvis plans the task, chooses which models it needs, has those models perform the task and then generates and issues a response. The workflow of this system consists of four stages:
- Task Planning: Using ChatGPT to analyze the requests of users to understand their intention, and disassemble them into possible solvable tasks via prompts.
- Model Selection: To solve the planned tasks, ChatGPT selects expert models that are hosted on Hugging Face based on model descriptions.
- Task Execution: Invoke and execute each selected model, and return the results to ChatGPT.
- Response Generation: Finally, using ChatGPT to integrate the prediction of all models, and generate answers for users.
HuggingGPT
YouTube ... Quora ...Google search ...Google News ...Bing News
- HuggingGPT | Microsoft ... A system to connect LLMs with ML community.
- How to Use Jarvis, Microsoft's One AI Bot to Rule Them All | Avram Piltch - Tom's Hardware
- How to Use Microsoft JARVIS (HuggingGPT) Right Now | Arjun Sha - Beebom
- HuggingGPT ... A Framework That Leverages LLMs to Connect Various AI Models in Machine Learning Communities Hugging Face to Solve AI Tasks
HuggingGPT that the Microsoft researchers have set up that leverages Large Language Model (LLM) such as ChatGPT to connect various AI models in machine learning communities to solve AI tasks. Specifically, HuggingGPT uses ChatGPT to conduct task planning when receiving a user request, select models according to their function descriptions available in Hugging Face, execute each subtask with the selected AI model, and summarize the response according to the execution results. To use HuggingGPT, you'll need to obtain an OpenAPI API Key if you don't already have one and sign up for a free account at Hugging Face. Once you've logged in to the site, navigate to Settings -> Access Tokens by clicking the links in the left rail.
Auto-GPT
YouTube ... Quora ...Google search ...Google News ...Bing News
- Auto-GPT | Significant Gravitas - GitHub
- GPT-4 | OpenAI
- ElevenLabs
- Pinecone
- Colaboratory | Google
- What is Auto-GPT? Everything to know about the next powerful AI tool | Sabrina Ortiz - ZDnet ... Auto-GPT can do a lot of things ChatGPT can't do.
- 6 Common Auto-GPT Installation Issues and How to Resolve Them | Jayric Maning - MakeUseOf
- AutoGPT: Everything You Need To Know | Nisha Arya - KDnuggets ... GPT has the ability to write its own code using GPT-4. It also executes Python scripts which allow it to recursively debug, develop, build and continuously self-improve.
- Revolutionizing Autonomous AI: Harnessing Vector Databases to Empower Auto-GPT | Sim Fu - zilliz ... Auto-GPT has limitations in understanding and retaining extensive contextual information because the GPT model it leverages has a token limit
Auto-GPT (AutoGPT) is an open-source Python AI Agent application based on GPT-4 that can self-prompt. This means that if the user states an end goal, the system can work out the steps needed to get there and carry them out. Auto-GPT works by setting a goal; the AI will then generate and complete tasks. Basically, it does all the follow-up work for you, asking and answering its own prompts in a loop. It utilizes the GPT-4 API and can perform a task with little human intervention.
Auto-GPT manages short-term and long-term memory by writing to and reading from databases and files; manages context window length requirements with summarization; can perform internet-based actions such as web searching, web form, and API interactions unattended; and includes text-to-speech for voice output. However, it has limitations in understanding and retaining extensive contextual information because the GPT model it leverages has a token limit. One way to address this contextual issue is to access a window of historical messages, such as the last ten messages or a fixed number of tokens, without exceeding the token limit of a single conversation. However, this method restricts Auto-GPT from accessing earlier contextual information, which might lead to the failure of Auto-GPT to accomplish its goal
To install Auto-GPT, you will need to have Python and Pip installed on your computer. You can download the latest version of Python from the official website and install it on your computer. You will also need to add API keys to use Auto-GPT. You can go to the GitHub release page of Auto-GPT and download the ZIP file by clicking on “Source code (zip)”
If you want Auto-GPT to speak using ElevenLabs, you will need to have an ElevenLabs API key. You can obtain your ElevenLabs API key from their website. Once you have your API key, you can add it to the .env file in the Auto-GPT directory.
Auto-GPT is not yet capable of achieving the AGI (Artificial General Intelligence) due to data quality, generalization, and explainability issues. - Kanwal Mehreen
Breaking Down AutoGPT | Kanwal Mehreen - KDnuggets ... here are the steps
- Input from the User
- Task Creation Agent
- Task Prioritization Agent
- Communication Between Agents
- Final Result - It also uses external memory to keep track of history and learn from its past experiences to generate more precise results.
The actions of these agents are visible on the user end in the following form:
- Thoughts: AI agent share their thoughts after completing the action
- Reasoning: It explains its choices of why is it choosing a particular course of action
- Plan: The plan includes the new set of tasks
- Criticism: Critically review the choices by identifying the limitations or concerns
Reflexion
YouTube ... Quora ...Google search ...Google News ...Bing News
- Reflexion: an autonomous agent with dynamic memory and self-reflection | N. Shinn, B. Labash, A. Gopinath - arXiv
- GPT-4 | OpenAI
- AlfWorld
Reflexion is a meta-technique approach that endows an agent with dynamic memory and self-reflection capabilities to enhance its existing reasoning trace and task-specific action choice abilities1 It builds on recent research and allows agents to learn from their mistakes and solve novel problems efficiently through a process of trial and error. Reflexion’s success has been demonstrated through evaluations in AlfWorld and HotPotQA environments, achieving success rates of 97% and 51%, respectively. To achieve full automation, Reflexion introduces a straightforward yet effective heuristic that enables the agent to pinpoint hallucination instances, avoid repetition in action sequences, and, in some environments, construct an internal memory map of the given environment.
Self-reflection allows humans to efficiently solve novel problems through a process of trial and error.
Autonomous GPT
YouTube ... Quora ...Google search ...Google News ...Bing News
- Embedding ... Fine-tuning ... RAG ... Search ... Clustering ... Recommendation ... Anomaly Detection ... Classification ... Dimensional Reduction. ...find outliers
- Developers Are Connecting Multiple AI Agents to Make More ‘Autonomous’ AI | Chloe Xiang - Vice ... Auto-GPT
- Auto-GPT | Toran Bruce Richards ... driven by GPT-4, chains together LLM "thoughts", to autonomously achieve whatever goal you set by can prompting itself to complete an objective.
- Task-driven Autonomous Agent Utilizing GPT-4, Pinecone, and LangChain for Diverse Applications | Yohei Nakajima
AgentGPT
YouTube ... Quora ...Google search ...Google News ...Bing News
- AgentGPT
- Beyond ChatGPT: AgentGPT is Bringing Autonomous AI To The Browser NOW | Sebastian - Medium
- AgentGPT template | Vercel ... Assemble, configure, and deploy autonomous AI Agents in your browser, using LangChain, OpenAI, AutoGPT and T3 Stack
AgentGPT allows you to configure and deploy Autonomous AI agents. Name your custom AI and have it embark on any goal imaginable. It will attempt to reach the goal by thinking of tasks to do, executing them, and learning from the results
BabyAGI
YouTube ... Quora ...Google search ...Google News ...Bing News
- Auto-GPT and BabyAGI: How ‘autonomous agents’ are bringing generative AI to the masses | Mark Sullivan - Fast Company ... Autonomous agents may mark an important step toward a world where AI-driven systems are smart enough to work on their own, without need of human involvement.
- AI & Identity, from East & West, with Yohei Nakajima GP at Untapped Capital and BabyAGI Creator | Erik Torenberg and Nathan Labenz - The Cognitive Revolution
OpenAGI
- OpenAGI: When LLM Meets Domain Experts | Y. Ge, W. Hua, K. Mei, J. Ji, J. Tan, S. Xu, Z. Li, Y. Zhang | Arxiv
- OpenAGI | Github
OpenAGI is an open-source research platform for artificial general intelligence (AGI). It's designed to offer complex, multi-step tasks. OpenAGI offers complex tasks, datasets, metrics, and models for solving tasks. It also provides task-specific datasets, evaluation metrics, and a diverse range of extensible models. OpenAGI uses a dual strategy, integrating standard benchmark tasks for benchmarking and evaluation, and open-ended tasks including more expandable models, tools, plugins, or APIs for creative problem-solving. OpenAGI formulates complex tasks as natural language queries, serving as input to the LLM. It also proposes an LLM+RLTF approach to learning better task design.
AutoGen
YouTube ... Quora ...Google search ...Google News ...Bing News
- AutoGen | Microsoft
- AutoGen Studio 2.0: Revolutionizing AI Agents
- Create AI Agents Easily with AutoGen Studio UI 2.0
- AutoGen Studio UI 2.0 : Step By Step Installation Guide
- AutoGen Studio: Interactively Explore Multi-Agent Workflows
AutoGen Studio 2.0** is a comprehensive tool suitable for developers of all skill levels. It simplifies AI development by providing an intuitive interface and extensive toolset. The platform caters to a broad spectrum of developers, from novices to experts.
Getting Started:
- Environment Preparation: Crucial steps include installing **Python** and **Anaconda**.
- Configuring LLM Provider: Obtain an API key from **OpenAI** or **Azure** for language model access.
- Installation and Launch: A simplified process to kickstart AutoGen Studio.
- Interface Navigation:
- Build Section: Enables AI agent creation, skill definition, and workflow setup.
- Playground Section: A dynamic platform for testing and observing AI agent behavior.
- Gallery Section: Stores AI development sessions for future reference.
- Powerful Python API:
- Beneath its web interface, AutoGen Studio features a **Python API**.
- Developers can use this API to gain detailed control over agent workflows.
MultiOn
YouTube ... Quora ...Google search ...Google News ...Bing News
Personal AI agent and life copilot that uses the browser to execute complex tasks.
- MultiOn Browser is a web browser that uses ChatGPT and OpenAI plugins to interact with anything on the internet on your behalf
- MultiOn is also a ChatGPT plugin that can automate tasks for you, such as posting on social media or ordering products online, or find any content on the web
Agents with Reinforcement Learning (RL)
Reinforcement Learning (RL) aims to make an agent (our “model”) learn through the interaction with an environment (this can be either virtual or real). RL was firstly developed to adhere to Markov Decision Process (MDP)es. In this ambit, an agent is placed in a stochastic stationary environment and tries to learn a policy through a reward/punishment mechanism. In this scenario, it is proved the agent will converge to a satisfactory policy. However, if multiple agents are placed in the same environment, this condition is no longer true. In fact, before the learning of the agent was only dependent on the interaction between the agent and the environment, now it is also dependent on the interaction between agents
AI-Powered Search
YouTube ... Quora ...Google search ...Google News ...Bing News
- Embedding ... Fine-tuning ... RAG ... Search ... Clustering ... Recommendation ... Anomaly Detection ... Classification ... Dimensional Reduction. ...find outliers
- Excel ... Documents ... Database; Vector & Relational ... Graph ... LlamaIndex
- Pinecone
- Recurrent Neural Network (RNN)
- Autoencoder (AE) / Encoder-Decoder
Robotic Process Automation (RPA)
Robotic process automation (RPA) is a software technology that allows businesses to automate repetitive, rule-based tasks. RPA software robots, or "bots," can mimic human actions by interacting with computer systems and software applications. This can free up human employees to focus on more strategic and value-added tasks. RPA is a powerful tool that can be used to automate a wide variety of tasks, including:
- Data entry
- Data extraction
- Data validation
- Report generation
- Email management
- Workflow automation
- Compliance checks
- Customer service
- IT support
AI is being used with RPA in a number of ways, including:
- Automating tasks that require human judgment: AI can be used to automate tasks that require human judgment, such as identifying fraud or making predictions. This can free up human employees to focus on more strategic and value-added tasks.
- Making RPA more efficient: AI can be used to make RPA more efficient by automating tasks that are currently done manually, such as data extraction and processing. This can save time and resources, and it can also help to ensure that tasks are done accurately and consistently.
- Improving the accuracy of RPA: AI can be used to improve the accuracy of RPA by identifying and correcting errors. This can help to ensure that RPA is producing accurate and reliable results.
- Expanding the capabilities of RPA: AI can be used to expand the capabilities of RPA by enabling it to perform tasks that were previously not possible, such as understanding natural language and making decisions based on incomplete or uncertain information. This can make RPA a more powerful tool for automating a wider range of tasks.
Tools
Jane Fulton Suri defines in her book as “Thoughtless Acts” actions that we do without thinking to adapt, exploit, and react to things in our environment. Those actions underline unconscious behavior that are used to comply with a world not perfectly suited to our needs.
IFTTT
- IFTTT: If This Then That ... automating over 900 popular apps
IFTTT's AI tools take your existing automations further, generating creative content like social media posts or blog outlines, summarizing key points, and even crafting witty responses to prompts. Think of it as adding a smart assistant to your routines, saving you time and effort while adding a touch of AI magic to your automated workflows.
- Smart Automation:
- AI-Sync Integrations: Control smart home devices like fans and lights based on triggers from other systems like Ecobee or voice assistants.
- Predictive Automations: Trigger actions based on anticipated events, like sending a birthday message before the day even arrives.
- Personalized Workflows: Tailor your automations to your specific needs and preferences using AI-powered insights and suggestions.
- Content Creation:
- AI Content Creator: Generate blog drafts, outlines, social media posts, and even video scripts based on keywords or prompts.
- AI Summarizer: Condense articles, emails, or meeting notes into concise, easy-to-understand summaries.
- AI Social Creator: Craft witty and engaging replies to social media mentions, saving you time and effort.
- Data Analysis:
- AI-powered Triggers: Set triggers based on extracted data points, like stock prices reaching a certain threshold or changes in weather conditions.
- Automatic Insights: Generate reports and summaries from connected data sources, providing you with actionable information.
- Pattern Recognition: Identify trends and patterns in your data to automate future actions or optimize existing workflows.
OutSystems
OutSystems infuses AI throughout its platform, offering pre-built components and AI-powered suggestions to simplify building intelligent apps, from code analysis to generating content based on user input.
- AI Assist: Offers intelligent recommendations for data models, UI elements, and integration points, accelerating development.
- AI Hub: Provides pre-built AI components like sentiment analysis, image classification, and anomaly detection, easily integrated into applications.
- Virtual AI Assistant integration: Enables building chatbots and voice assistants within applications.
Appian
- Appian ... Artificial Intelligence
Appian offers pre-built AI skills and low-code tools to seamlessly integrate AI capabilities like document understanding, image recognition, and even text generation into your business applications, transforming them into intelligent decision-making engines.
- Intelligent Document Processing (IDP): Appian's IDP uses machine learning to automatically extract and analyze data from documents like invoices, contracts, and forms. This eliminates manual data entry and streamlines data capture within process automation workflows.
- Predictive Analytics: Appian's AI models can predict future events and trends based on historical data. This enables proactive interventions to prevent issues before they arise and optimize resource allocation within processes.
- Decision Modeling: Appian provides tools for building and deploying decision models that automate complex decisions based on pre-defined rules and machine learning models. This improves decision consistency and efficiency within your processes.
- AI Copilot: Appian's AI Copilot is a design assistant that leverages machine learning to suggest optimal process design elements, identify potential bottlenecks, and recommend improvements. This accelerates process development and optimizes efficiency.
- Integration with external AI services: Appian can integrate with various AI services like Google Cloud AI, Microsoft Azure AI, and Amazon SageMaker, expanding its capabilities and allowing you to leverage specific AI features within your automation workflows.
Power Apps (Microsoft)
Power Apps uses AI through AI Copilot, which helps build apps by understanding natural language descriptions, and AI Builder, which adds pre-trained AI models for tasks like sentiment analysis or text extraction directly within your apps.
- AI Builder: Offers pre-built AI models for tasks like sentiment analysis, image recognition, and text classification.
- Power Automate: Integrates with Azure AI services for more advanced AI capabilities.
- Cognitive Services: Provides access to a wide range of Azure AI services like speech recognition, translation, and computer vision.
Salesforce Platform
Salesforce infuses AI through its "Einstein" suite, offering pre-built tools like predictive lead scoring and chatbot assistants to automate tasks, gain data insights, and personalize customer experiences.
- Einstein AI: Salesforce's native AI platform offering pre-built AI models for tasks like lead scoring, anomaly detection, and customer sentiment analysis.
- Predictive scoring and recommendations: Uses AI to predict customer behavior and recommend personalized actions.
- Natural Language Processing (NLP): Enables building chatbots and other applications that understand and respond to natural language.
Mendix
Offers AI-powered features like chatbots and anomaly detection, but with less focus than other leaders.
- AI-powered microflows and nanoflows: building blocks for automation, microflows and nanoflows, can be enhanced with AI components like decision trees, machine learning models, and pre-built AI connectors. This allows you to automate complex decisions and tasks within your processes.
- Intelligent document processing (IDP): IDP capabilities use AI to automatically extract data from documents like invoices, contracts, and forms. This eliminates manual data entry and streamlines data capture within process automation workflows.
- Predictive analytics and recommendations: offers pre-built AI models and connectors for tasks like anomaly detection, predictive maintenance, and next-best-action recommendations. This empowers you to anticipate issues, optimize resource allocation, and personalize user experiences within your processes.
- Chatbots and virtual assistants: allows you to build AI-powered chatbots and virtual assistants that can automate routine tasks, answer user questions, and guide users through processes. This improves user experience and reduces workload for human agents.
- Low-code integration with AI services: seamlessly integrates with various AI services like Google Cloud AI, Azure AI, and Amazon SageMaker. This opens up a vast range of AI capabilities for your process automation needs.
Retool
Primarily focused on developer tools, but offers limited AI capabilities like data visualization and anomaly detection.
- Real-time data visualization and anomaly detection: AI features can analyze data streams from your processes in real-time, identify anomalies, and trigger alerts to notify your team. This helps you proactively catch issues before they escalate.
- Automated data transformations: allows you to build custom data workflows using JavaScript and integrate AI libraries for tasks like data cleaning, normalization, and feature engineering. This empowers you to prepare and analyze data for process optimization.
- Machine learning model integration: lets you connect your own machine learning models or leverage pre-built AI services to perform tasks like predictions, recommendations, and sentiment analysis within your process automation workflows.
- API-driven automation: focus on APIs allows you to automate interactions with various tools and services involved in your processes, streamlining data flow and triggering actions based on your AI analysis.
ServiceNow
ServiceNow embeds AI across its platform, from virtual agents answering customer queries to predictive models optimizing workflows, boosting employee and customer experiences with intelligent automation.
- Process Mining: ServiceNow AI analyzes past activity logs to identify inefficiencies, bottlenecks, and opportunities for improvement within existing processes.
- Intelligent Routing: ServiceNow AI can dynamically route tasks and cases based on real-time data like workload, skillsets, and urgency.
- Predictive Analytics: ServiceNow's AI models predict future events and trends based on historical data. This enables proactive interventions to prevent issues before they arise and improve the overall quality of service.
- Automated Decision Making: ServiceNow AI can automate routine decisions based on pre-defined rules and machine learning models.
- Integration with Now Workflow: ServiceNow AI seamlessly integrates with the Now Workflow engine, allowing you to easily build automated workflows that leverage AI capabilities. This simplifies implementation and provides a unified platform for process automation.
UiPath
In UiPath, AI works alongside RPA to create Intelligent Process Automation (IPA), significantly enhancing the capabilities of robotic automation.
- UiPath AI Computer Vision: Recognizes objects and text within applications and documents, enabling robots to interact with dynamic interfaces.
- UiPath Document Understanding: Extracts and structures data from documents, powering automated document processing.
- UiPath Machine Learning: Builds and deploys custom ML models within robots for specific tasks like anomaly detection or sentiment analysis.
- UiPath AI Fabric: Integrates various AI tools and services into a unified platform for streamlined development and deployment of AI-powered automation.
Multi-Agent
- Towards the AI Agent Ecosystem | M. Wu, J. Matheson, J. Stener and A. Steele ... A Technical Guide for Founders & Operators Building Agents
Multi-Agent Communications
Youtube search... ...Google search
- Telecommunications ... Computer Networks ... 5G ... Satellite Communications ... Quantum Communications ... Communication Agents ... Smart Cities ... Digital Twin ... Internet of Things (IoT)
- Attention
- TALISMAN: A multi-agent system for natural language processing | Marie-Hélène Stefanini & Yves Demazeau - Part of the Lecture Notes in Computer Science book series (LNAI,volume 991) ... One of the originalities of this system is the distributed treatment of sentence analysis (as opposed to a classic sequential treatment) and the introduction of linguistic laws which handle the communication between agents, without central control. At the implementation level, the system brings openness to dictionary modification, grammars and strategies of analysis, as well as the necessary mechanisms for the integration of new modules.
- Integrating AI Planning with Natural Language Processing: A Combination of Explicit and Tacit Knowledge | Kebing Jin, Hankz Hankui Zhuo - Cornell University
- BLIP: Bootstrapping Language-Image Pre-training for Unified Vision-Language Understanding and Generation | J. Li, S. Hoi, and D. Rose - Salesforce AI Research
[bots] all communicating with other bots, but we find that you know it doesn't really make sense for these bots to like reduce everything to language and then send the language over and then have it be you know kind of re-embedded. Like why don't they just talk to each other in their native language which is this high dimensional space. ... you can adapt embeddings to another, you know, embedding space with basically just like a single of your projection. - Nathan Labenz | The Cognitive Revolution; 'Looking Up the AI Exponential with Azeem Azhar of the Exponential View episode'
Multi-Agents Reinforcement Learning (MARL)
In a similar vein, multi-agent RL also addresses sequential decision-making problems, but with more than one agent involved. In particular, both the evolution of the system state and the reward received by each agent are influenced by the joint actions of all agents. More intriguingly, each agent has its own long-term reward to optimize, which now becomes a function of the policies of all other agents.
- Markov/Stochastic Games
- Cooperative Setting
- Competitive Setting
- Mixed Setting
- Extensive-Form Games
Challenges
Despite a general model with broad applications, MARL suffers from several challenges in theoretical analysis, in addition to those that arise in single-agent RL.
- Non-Unique Learning Goals - Unlike single-agent RL, where the goal of the agent is to maximize the long-term return efficiently, the learning goals of MARL can be vague at times. ...Indeed, the goals that need to be considered in the analysis of MARL algorithms can be multi-dimensional ... is undoubtedly a reasonable solution concept in game theory, under the assumption that the agents are all rational, and are capable of perfectly reasoning and infinite mutual modeling of agents. However, with bounded rationality, the agents may only be able to perform finite mutual modeling
- Non-Stationarity - multiple agents usually learn concurrently, causing the environment faced by each individual agent to be non-stationary. In particular, the action taken by one agent affects the reward of other opponent agents, and the evolution of the state. As a result, the learning agent is required to account for how the other agents behave and adapt to the joint behavior accordingly. This invalidates the stationarity assumption for establishing the convergence of single-agent RL algorithms
- Scalability Issue - To handle non-stationarity, each individual agent may need to account for the joint action space, whose dimension increases exponentially with the number of agents. This is also referred to as the combinatorial nature of MARL
- Various Information Structures - Compared to the single-agent case, the information structure of MARL, namely, who knows what at the training and execution, is more involved. For example, in the framework of Markov games, it suffices to observe the instantaneous state st , in order for each agent to make decisions